Chaoran Cheng
Siebel School of Computing and Data Science, University of Illinois Urbana-Champaign
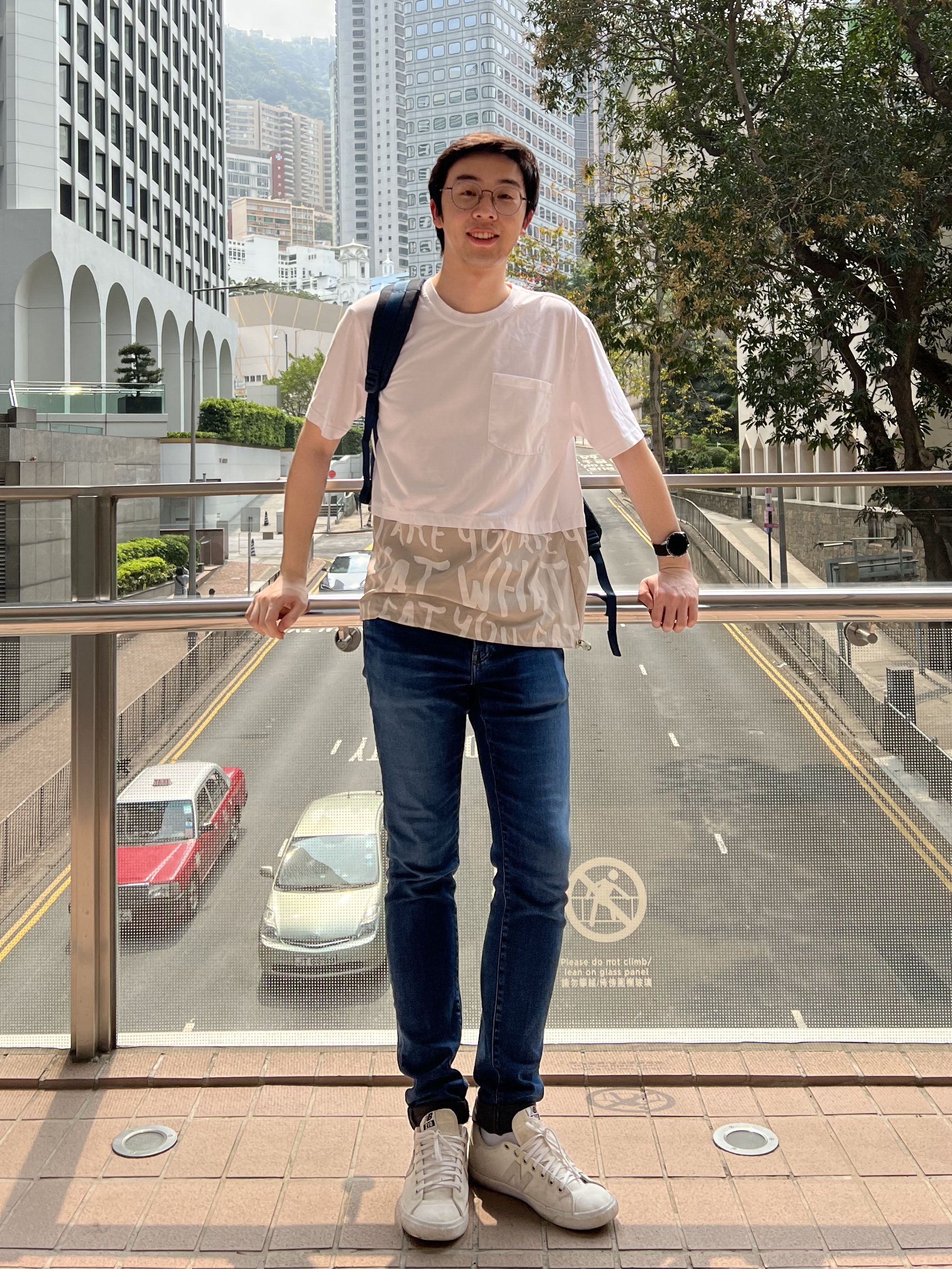
Hi! I am Chaoran Cheng, a third-year CS Ph.D. candidate at the University of Illinois Urbana-Champaign. I am advised by Prof. Ge Liu, before which I was working with Prof. Jian Peng. My research interest mainly spans multiple AI4Science topics with a special focus on generative tasks on 3D molecules and proteins including sequence-structure co-design and structure optimization. Combining both the continuous and discrete modalities together, our work can build a more comprehensive and versatile generative model for molecules and proteins on diverse downstream tasks. I am actively engaged in the MMLI project, a joint effort between UIUC CS School and Chemistry Department to develop a machine learning-based platform for molecular design.
Out of my pure personal interest, I am developing a machine-learning based automatic charter for Cytoid, a community-driven rhythm game inspired by Rayark’s games Cytus and Cytus II. The community provides many fan-made charts that can be used to train a machine learning system.
I obtained my Bachelor’s Degree in EECS, Peking University, before which I also spent my freshman year studying chemistry.
News
Oct 7, 2024 | My contributing work on AI2BMD during my internship at MSRA has been accepted to Nature. |
---|---|
Sep 27, 2024 | Our latest work of Statistical Flow Matching (SFM) for discrete generation has been accepted to NeurIPS 2024! Check out our paper on arXiv and our code on GitHub. We applied SFM across image, text, and biological domains demonstrate its superior sampling quality and NLL on discrete tasks.
|
Sep 21, 2023 | My work Equivariant Neural Operator Learning with Graphon Convolution was accepted to NeurIPS 2023 as a Spotlight paper. Check out the paper here. I am also honored to be the Workflow Chair of the New Frontiers in Graph Learning Workshop at NeurIPS 2023! |
Dec 7, 2022 | Check out this post on the Tensor Field Network! ⌬ ![]() |
Nov 30, 2022 | Get a glimpse of Cézanne and the revolution of modern art. ![]() |